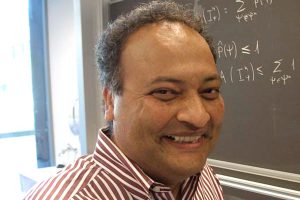
Professor
Vwani Roychowdhury
AD
Most Helpful Review
Fall 2018 - For a supposedly simple class the professor made it very difficult. Lectures were not clear and have the class didn’t show up. He gave one more homework than on the syllabus plus the project was only a week long instead of the planned 3 weeks so instead of studying for finals everyone was trying to write the long lines of code needed for the vague project spec. Only good thing, nice grading.
Fall 2018 - For a supposedly simple class the professor made it very difficult. Lectures were not clear and have the class didn’t show up. He gave one more homework than on the syllabus plus the project was only a week long instead of the planned 3 weeks so instead of studying for finals everyone was trying to write the long lines of code needed for the vague project spec. Only good thing, nice grading.